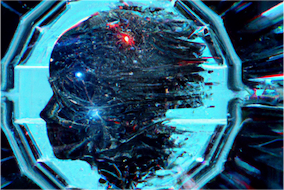
Causal Emergence
Complex systems, like living organisms, environments, health, socioeconomics, or online communities, are unified entities composed of numerous interacting units. Grasping the emergence and evolution of their causal structures is essential. We utilize advanced machine learning technologies, such as causal representation and reinforcement learning, and information theory methods, including information decomposition, to identify emerging causal structures and predict future evolutionary states.